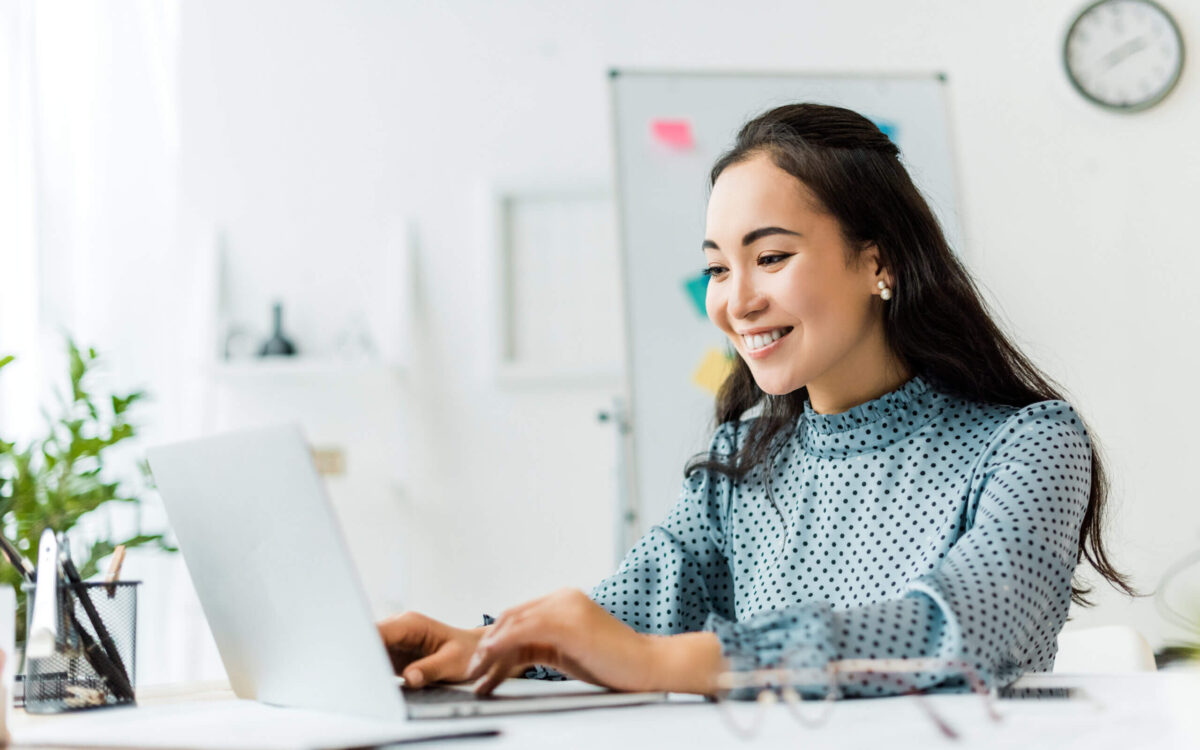
How to Use Data (And Your Instincts) to Evaluate Your Next AI Project
Don’t get me wrong – I am genuinely optimistic about AI and math modeling in general. Our world is constantly changing because of it.
I’ve seen some incredible contact center AI ideas, and have seen some fantastic results. But, I’ve also heard of some poor projects, and I understand many of the pitfalls.
I want to help you avoid these perils and bypass AI blunders.
We contact center software vendors have a duty to our customers.
I’ve been lucky enough to have worked for some very just companies, where the guiding principle in decision-making is to “do what is right.” But complex projects are hard on a vendor, too, and oftentimes there is a temptation to minimize their own risk when proving out new technologies.
In other words, contact center vendors sometimes cut corners when it comes to big, expensive AI projects in order to show the “results” they were hoping to achieve.
Here are a few lessons I’ve picked up along the way that you can use to evaluate your next AI project.
Follow your gut.
It’s important to ask yourself: is there truly a problem that needs to be solved? Or, are you being sold a solution to a problem that you’re not so sure you have?
And then, when it comes to the solution – does it all add up? Meaning, if you’re being thrown a ball of jargon from the vendor that you don’t understand and it isn’t being explained to you, you might want to throw up the red flag. Sometimes vendors will serve you technobabble and not explain the actual solution until you get it. You might feel uninformed, but trust me, It’s entirely possible your vendor doesn’t understand their buzzwords either.
On the same note, if a model, or a complex system produces counterintuitive results, watch out. Your vendor should be able to explain to you, in simple terms, how the models work and what they find. They should not be trying to blind you with science.
How is the AI part going?
In an AI exercise, if the vendor keeps asking for more and more data, that is a red flag. It basically means that the lab-version of their model, with your data, is not seeing results.
Start asking for model validation graphs on contact center performance metrics. Be wary of “statistics-speak”. The lift should be obvious to your own eyes.
Keep perspective.
When boiled down, AI is simply another math technique. Sometimes better, sometimes worse than other math techniques.
And, if you take a closer look, you’ll see that some of the “new” automated forecasters are using old-timey, non-AI math. Don’t assume there is any value to something just because we vendors call it AI.
Teach your data scientist.
A shocking thing to find out is that most of the folks doing the hard work–the behind the scenes model building–probably know little about your business.
Big companies tend to use product managers to understand the business, while the data scientists do the work. This is a failure point in a big project.
I spoke to a data science group once whose members had never been in a contact center. They didn’t even know the basics. One said to me incredulously: “Did you know that agents quit a lot, and that attrition is high? We have to rewrite all of our models every time one quits!”
Give your data scientists a tour of your contact center and make sure that they are visible during the project. The product manager wall between the engineers is a very weak link.
It’s the data scientists’ responsibility to solve real problems for our customers. If we have a new cool idea, we better be able to back our innovation up with real, provable, and obvious ROI in your operations.
Listen for excuses.
A sure sign of imminent failure is when the vendor starts questioning your operation, your data, or your management. When you hear that the “data is not clean”, blame is starting to be assigned to you.
If you’re promised ROI, and not seeing that ROI, you should be able to get your money back. I’m lucky to work for a very ethical CEO. He’s let me know that we’d return our customer’s money – all of it – if we couldn’t find ROI. We shouldn’t waste our customer’s money if a project isn’t working. And that should be the industry gold standard.
Your gut can make your AI project more successful.
As a customer, it’s important to challenge the vendor, their premise, and their understanding of your business problem.
When investing both your money and your time, it’s crucial that you be critical of the vendor (as well as a good team player). A good vendor should always welcome some skepticism.
AI systems are like any other math technique. Some are good, and some are bad. Even though AI is at the height of fashion right now, it too will go out of style (big data or six sigma, anyone?).
And while algorithms are increasingly being called AI (whether they are or not) we should be able to call BS on AI, just like we would on any other technology.